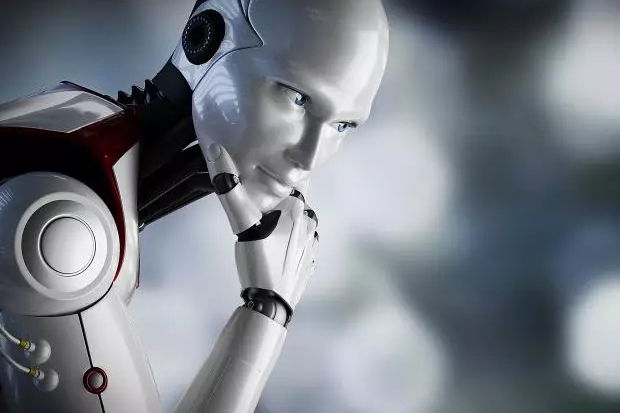
This article is a re-post from Fathom Consulting's Thank Fathom its Friday: "A lighthearted look at the week's events". It is here with permission
by Oliver White*
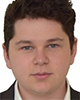
Wind the clock back 80 years and Colossus, one of the first electronic computers, whose name was fitting of its size, was built during the second world war to decrypt secret German codes. Almost inconceivably, it used vacuum tubes and paper tape to perform several basic Boolean (e.g. true/false) logical operations.
But fast forward to 2018 and not only do we carry them around in our pockets, but computers are beginning to 'think' for themselves and even modify their own core code base - essentially become artificially intelligent. Artificial intelligence (AI) is the degree to which computer systems can sense their environment, 'think' for themselves, learn and then take actions as a result. Such ability to alter behaviour based on a changing environment separates artificial intelligence distinctly from just the pure automation of routine tasks.
How far computers and AI as a technology have come can be highlighted in a nutshell by one piece of software: AlphaGo, an AI computer programme built by Google's DeepMind corporation that plays the board game Go. There's a very good reason why the game of Go was chosen to be part of this project. Go is considered much more difficult for computers to win compared to other games such as chess, because it inherently has much larger branches of possible moves (approximately 300 times the number of chess). This means traditional AI methods struggle to compute the best possible play at any given time.
However, in October 2015, AlphaGo became the first computer programme to beat a human professional Go player without handicaps, and in 2017, AlphaGo beat Ke Jie, the world number one player at the time, in a three-game match. Such a feat had been considered impossible by experts in the field.
But how can AI be applied to the world of economic research?
Being in the economic forecasting game, as we are here at Fathom Consulting, the topic of pioneering the next generation of economic models based on artificial intelligence has been the subject of many discussions. The idea of an economic model that improves based on scenarios we feed into it, becoming more accurate, adapting to changes in input data and learning users' behaviour to tailor output specific to them fills us all with excitement, and is surely the future. If this materialises, then the role of an economist will undoubtedly be enhanced, but the actual number of economist jobs that will be replaced entirely by the AI uprising is less clear.
Something we at Fathom like to pride ourselves on is the fact that we produce rigorous analysis and 'think' about the work we are doing while applying human logic to problem-solving. While modern AI programmers would like to believe that strides are being made towards this becoming a reality, artificial intelligence software is still some way off being able to 'think' like a human. Essentially, it's an argument of comparative advantage, where humans 'trade' skills with AI in a mutually beneficial way. Humans will always have a comparative advantage over AI in understanding other humans, even if one day we lose the absolute advantage.
Ultimately, the goal of artificial intelligence is for a computer to be able to solve problems in a similar way to a hypothesised human brain, taking the same logical steps and applying similar human intuition. Alan Turing's test of a machine's ability to exhibit intelligent behaviour equivalent to, or indistinguishable from, that of a human has already been passed by AI, and one assumes that it wouldn't be a huge leap for it to be able to mimic human intuition. That said, even the most cutting-edge artificial intelligence systems available today typically work with problems containing levels of complexity several orders of magnitude lower than the human brain, closer to the computing power of a worm. This not only shows how complex the human brain is, but also the fact that there's still a long way to go!
But, as economists, should we be worried?
Before we get too deep into the doom and gloom, it's worth stressing that AI isn't, and needn't be, synonymous with job losses. It's probable that a large proportion of jobs themselves won't entirely vanish; rather, they'll be redefined. Nevertheless, there are some exceptions. Driverless cars will put taxi drivers out of work. Full stop. No redefining taking place there. However, in a recent note, we dug a little deeper and found that it's likely the jobs most at risk are ones which are on some level routine, repetitive or predictable. Ones that require little or no 'thinking' or creativity.
Frey and Osborne's 2013 paper ('The future of employment: how susceptible are jobs to computerisation?') assigned probabilities to the share of jobs in each industry in the US that were subject to automation. The chart below plots those probabilities along with the current average hourly earnings in those sectors and indicates that the majority of low-paying jobs remain at risk of automation.
By this logic, economist positions, on the surface at least, seem fairly safe (phew). Ours should be one of the last industries to be impacted by the rise of AI and automation, with more low-skilled, low-pay jobs facing the chop first.
So where does this all leave us?
The endgame of AI remains uncertain. But one thing is for sure, machines will continue to become ever smarter, performing tasks with ever more efficiency. As it currently stands, humans are empathetic, AI is not. Will this always be the case? Almost certainly not. And machine traders, displaying no human emotion, or never being swayed by bias or other 'human' reactions to events should be beneficial.
However, the scope for more cooperation with human workers is considerable. That AI should become a supplementary tool rather than a complete replacement surely seems like the more desirable option. Computers allow users to manipulate large datasets in a split second, but human insight is needed to leverage this to add value to research. Economists use both human intuition and considerable technical knowledge to carry out their duties, something AI hasn't mastered yet.
Either way, we all need to prepare ourselves for the next chapter in the AI revolution, but economists probably don't need to consider job-retraining schemes just yet.
This article is a re-post from Fathom Consulting's Thank Fathom its Friday: "A lighthearted look at the week's events". It is here with permission
7 Comments
I would have thought that economical forecasting would be one of the least appropriate fields for the application of artificial intelligence.
No doubt there are clunky basic computer programs in existence already. But to automate it further I'd say that there are too many variables. Too high a probability of unexpected influences coming into play where there would be subtle differences between what happened when they cropped up last time and now.
If it was used there would have to be a long list of disclaimers: This forecast is made based upon the following assumptions: a).... b)......c).....
Take for example certain economists on this site where 5 years ago they (he) was saying that Ak house prices would crash in the near future. That was a human who was using professional training plus gut instinct. Presumably something like a human version of AI. It was dead wrong. If he had written an AI program at that time, the program would have produced the wrong results (because it would have been tweaked to provide the desired outcome at that time). But who knows, that same program might produce the right answers if it was to be used right now.
Ideally AI should be put to much better uses than focusing on replacing expensive human wages. There are quite few industries that would benefit from improving the way we carry out certain large scale tasks, and therefore help us to lessen and hopefully avoid negative environmental impacts.
Take the Commercial Fishing Industry for example. AI can be use to improve the way we target and harvest certain types of species such as tuna. Where we could easily eliminate the use of nets and replace them with an automated pole line fishing system, which is far more environmentally friendly since it avoids bycatches and drift nets. An on board AI reporting system could be far more effectively regulate and adhere to fishing quotas.
Apparently NZ is already having to enforce a 'bycatch' monitoring system for all fishing vessels, under the government-planned Integrated Electronic Monitoring and Reporting System (IEMRS), so every vessel will eventually be equipped with monitoring cameras to verify bycatch rates.
BBC article: New Zealand debates access to dead sea life footage
http://www.bbc.com/news/world-asia-42729173
While machine learning and AI techniques in forecasting can be useful they are hardly correct. Often the markets move more due to sentiment and we do not have an accurate way of even describing a predictive model for that. Yes there have been papers on ways of measuring sentiment and the links to increases and decreases in value but neither have those solutions been accurate for assessing sentiment either. Much like describing the mechanics in trust for semantic web AI, to describing the human perceived market value in a predictive fashion, or even to driving down a normal street the AI is fraught with human errors & biases. Which is often the major problem and failure with AI and machine learning. Human biases and issues get coded straight into the projects. You can even easily trick AI and autonomous vehicles into failures quite easily with a humble sharpie pen. It would be even worse introduced to NZ roads with signs pointing down cliffs, missing or not even being able to identify the road median, and numerous right of way issues. In fact time and time again we have research proving the fallacies in thinking the AI is suitable or enough for control alone in complex and risky environments, (hundreds of people have lost lives because of it).
If you read the papers related to tricking the AI into thinking a cat is guacamole with adversarial images was the funniest. Sure turtle to rifle is an interesting safety measure issue, but I do not think a cat goes well with corn chips.
http://mashable.com/2017/11/02/mit-researchers-fool-google-ai-program/#…
We welcome your comments below. If you are not already registered, please register to comment.
Remember we welcome robust, respectful and insightful debate. We don't welcome abusive or defamatory comments and will de-register those repeatedly making such comments. Our current comment policy is here.