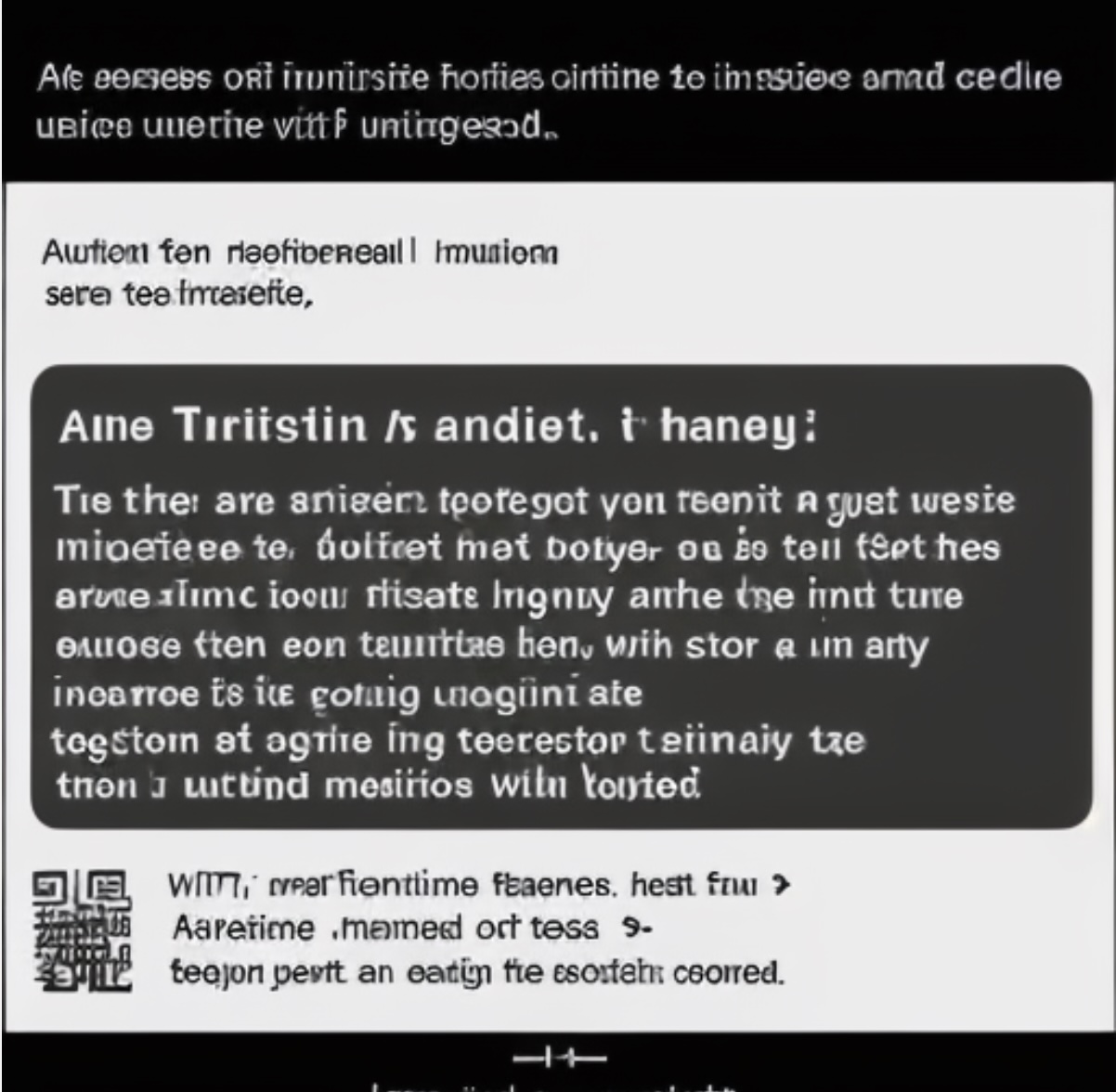
A quick note on one of the big use cases touted for generative artificial intelligence, namely summarising documents to extract the key points from them, to save time. Personally, I love that idea because I have to read such an awful lot and there's never enough time to go through a growing mountain of text.
Unfortunately, AI for summarising documents is a :sad trombone: (we really need to get some good emojis happening). "Worse than humans in every way" as Cam Wilson at Crikey put it.
This is what ASIC said it did:
"The potential for Generative Artificial Intelligence (Gen AI) technology is immense. As such, Australian Securities and Investments Commission (ASIC) sought to explore how these technologies work in practice with a real-life use case in the organisation.
ASIC procured AWS to run a Proof of Concept (PoC) between 15 January and 16 February 2024, to assess the capability of Gen AI Large Language Models (LLM) to summarise a sample of public submissions made to an external Parliamentary Joint Committee inquiry, looking into audit and consultancy firms.
The project team consisted of ASIC’s Chief Data and Analytics Office (CDAO) team, ASIC’s Regulatory Reform and Implementation team (who acted as subject matter experts) and AWS."
AWS is cloud computing giant Amazon Web Services which really does have a dog in the AI fight.
In summary, the assessors who weren't told which text was summarised by AI and which by humans overwhelmingly rated the living beings' work higher - 81 per cent to 47 per cent for the machines.
Section 5.1 in the PDF outlines the key themes from the analysis:
- Limited ability to pick up the nuance/context required to analyse submissions:
…it didn’t pick up the key issue in a nuanced way. I would have found it difficult to even use an output to craft a summary, I would just go back to original [submission].”
“The submission identified references to ASIC but it was wordy and pointless – just repeating what was in the submission“
- Included incorrect information in summaries:
“Included analysis which did not come from the document and does not serve the purpose. [Whereas] the human summary just said no references to ASIC.”
“Inaccurately raised legal professional privilege as a ‘conflicts of interest’ issue and repeated those considerations as references to more regulation of auditors/consultants.”
- Missed relevant information in summaries:
“Missed a lot of the commentary that was about ASIC (e.g. p4 content under the heading ‘corporate regulator’).“
For one assessor the AI missed where the submission had referred to external references which had recommendations in them.
- Missed the central point of submission:
“The summary does not highlight [FIRM]’s central point…”
“I would have expected summary to focus on 11 key points [outlined in submission], but didn’t see that level of detail.
- Focused on less relevant information (giving minor points prominence):
“Made strange choices about what to highlight.”
“Overall summary placed unnecessary emphasis on one minor recommendation around government procurement processes by opening with information on this, even though this recommendation was not the focus of either the inquiry or the [FIRM]’s submission.“
- Used irrelevant information from submission:
“A lot of extraneous information under the ‘references to ASIC’ subheading that is not about ASIC (directly or indirectly).
The AI picked up the content in attachment so included irrelevant information in listing recommendations (i.e. recommendations not from the submission itself). The assessor noted “this is not accurate and may cause misunderstanding.”
And so on - please note that no AI was used to summarise the assessment, just a tired old human who read it and copied over some of the information for the story, such as this pertinent bit:
Assessors generally agreed that the AI outputs could potentially create more work if used (in current state), due to the need to fact check outputs, or because the original source material actually presented information better.
The assessment used Meta's Llama 2 large language model, with 70 billion parameters - it's a big AI, in other words.
For the sake of completeness, I asked the newer Llama 3.1:70b model to summarise this story. It got most things right, bar this clanger which a non-techie might miss:
"The experiment involved using Amazon Web Services' large language model, Meta's Llama 2, with 70 billion parameters..."
Remember GIGO? Garbage In, Garbage Out, which is computer programming term to used to warn developers that you need to sanitise data before processing it. With GenAI, you risk Good Stuff In, Garbage Out. For now at least. People might not like the information they've provided treated that way. You're also left wondering how warped AI summaries of AI generated text might be.
Via David Gerard.
4 Comments
Not just public submissions.
I've reviewed (with others) what AI does to people's CVs and it is truly heartbreaking. But the recruitment 'professionals' (yeah, right) love it. "Such a game changer." Employers would do well to only employ recruiters that DO NOT use AI.
Agree for reference readers could refer Stanford University benchmarks, OpenAI GPT-4 has 0.96 mean score compared to 0.65 for Llama2 70b used in this case.
however, on legal documents most models don’t do well… see how much lower is the legal benchmark compared to average for any given model.
Prompt engineering also plays a big role in performance not just the model itself. Most models are fine tuned to follow human instructions in the form of text prompts.
Good thing they did was to setup an evaluation study but looks like it was setup to fail.
We welcome your comments below. If you are not already registered, please register to comment.
Remember we welcome robust, respectful and insightful debate. We don't welcome abusive or defamatory comments and will de-register those repeatedly making such comments. Our current comment policy is here.